In the past, data labels were applied manually. The manual labeling process is tedious and requires an individual to do each label. One business, for example, wanted to understand the feelings expressed by their clients in online reviews. Imagine your business needs to generate a data model based on 90,000 customer reviews. The labeler would need to work for 750 hours if he or she spent 30 seconds annotating each review.
Automated data annotation solutions have been developed in order to relieve enterprises of the tedious task of labeling, and to redirect them to their main goals. These solutions enable businesses to easily label thousands of pieces of information in seconds.
We will try to explain everything in this blog.
- What is Automated data Labeling (ADL)?
- How do auto-labels work?
- The Benefits of Automatic Data Labeling
- Key challenges to automated data labeling
Automatic Data Labeling: What Is it?
The term automatic labeling is used to describe data annotations performed by software instead of humans. Experts in data labeling develop AI which labels raw, unlabeled data. Human labelers identify and verify the label. The entire dataset is updated if the model labels the data successfully.
In some instances, however, the AI model may only work in one session, or it could label data inaccurately. Data will then be given to AI and trained again. This process continues until all data can be correctly labelled by the AI.
After the errors have been corrected and the data correctly labelled, they are added to the labeled collection for training. The accuracy with which the dataset is labeled determines if the model can be used to train another model. Using the labeled data, ML teams will train multiple models.
The human in the loop method is still important, even though the process of data labeling can be automated. machine learning Data labeling is critical to ensuring the quality and accuracy for machine-learning. Human labelers will manually review the annotations and fill in the gaps.
The Benefits of Automatic Data Labeling
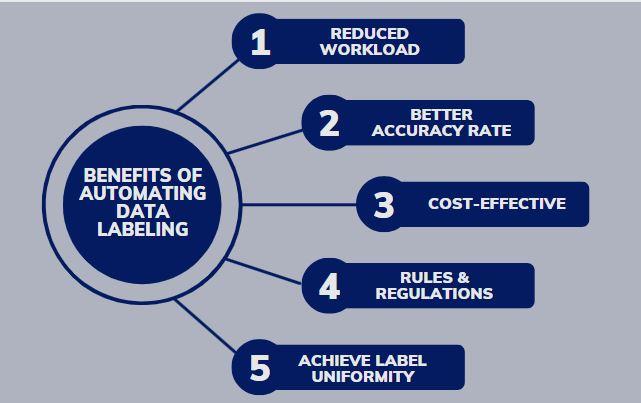
These are some benefits to choosing automated over manual labeling.
- Reducing Workload
Traditionally, when using manual labeling, an entire labeling team must manually label hundreds and even thousands of data each day. The process can last weeks, or even longer, depending on the amount of data. In the meantime, the company may collect more data. Businesses opt for automation to save both time and energy. Automation reduces the amount of human effort required for data annotation in a machine learning project. To train data, an auto-labeling system can be employed. Expert data labelers can edit or revise annotations in which the confidence rating is lower. The entire process requires less people and work.
- Improved Accuracy Rate
Active learning is a semisupervised technique that produces high accuracy data annotation. These data are trained and then tested to ensure accuracy. Businesses can avoid human errors. Automating data labeling keeps improving and improving your procedures.
- Cost-Effective
Some businesses still use the manual method of data labeling. This can lead to disruptions in operations, mistakes with labelling, or regulatory violations, which all increase costs for your business. Companies can reduce costs by using automated data annotation, which requires little or no human interaction. Further, it saves costs on the recruitment and hiring process.
- Rules & Regulations
Data security is governed by several laws, standards and guidelines. Cloud infrastructure is becoming more complex, increasing the number of threats and vulnerabilities. To reduce the dangers, new laws have been passed. Data compliance issues are complicated by the rapid change in standards and technologies. Automating compliance updates across your entire system is crucial as it allows you to track these changes continuously and ensures that your data always follows the rules and policies.
- Achieve Label Uniformity
Label uniformity is the biggest challenge that businesses face. When you manually label data, it is possible that different annotators, according to their own understanding, language, and culture, label these data – causing discrepancies. These data are not uniform and ineffective for AI/ML model training. A comprehensive model for auto-data labels could be beneficial. The tools have been pre-trained to help firms maintain consistency with their data labels.
Key challenges to automated data labeling
When a business is attempting to classify data, it faces a variety of challenges. Below are a couple of examples.
- Minimum Training Hours
Automation is superior to manual labeling but it still requires proper training. It is difficult to train the AI model. It is a challenging task for the annotator to train the AI model. Then, they must check its accuracy and re-train if needed. It takes a lot of time to prepare an AI model. Business houses can opt to use annotation services provided by a third party organization. They have data experts who can label the data correctly. Businesses can focus on their primary business goals.
- Irresistible with Multiple Use Cases
Models that are pre-trained have been designed specifically to produce a certain output based on the input data. A problem occurs when a company applies these models to data of a different type. The auto-labeling output may not be in line with the training cases of the model. Re-training an auto-labeling system to match the requirements of a project can take additional effort and time from the development team. For example, an auto-labeling system that is trained to label images in daylight, won’t be able label images at night.
- Manage Consistency
Objective and subjective data are both types of data.
- Objective data- True data or universal information is true regardless of the person who looks at it.
- Subjective data The same document can have different meanings depending on the person who reads it.
One of the most important components in evaluating data is to analyze how labels are defined. Even when using automation, different types of data may create confusion. For example, classifying a fruit as red is easy because the term is universal, but it becomes more difficult when dealing with complicated statistics. Companies can overcome this by using models that are trained to apply principles and regulations which remove the differences in data and give it a significant level of objectivity.
The conclusion of the article is:
Annotators often face a number of difficulties, and this is especially true when they are labeling manually. In order to accomplish these tedious tasks, it is important that humans and machines work together. The development of data annotation tools and techniques allows annotators to save time while labeling more data.
However, there are always some outsourcing opportunities. The data labeling services It is a good option for you to get high-quality information that suits your needs.